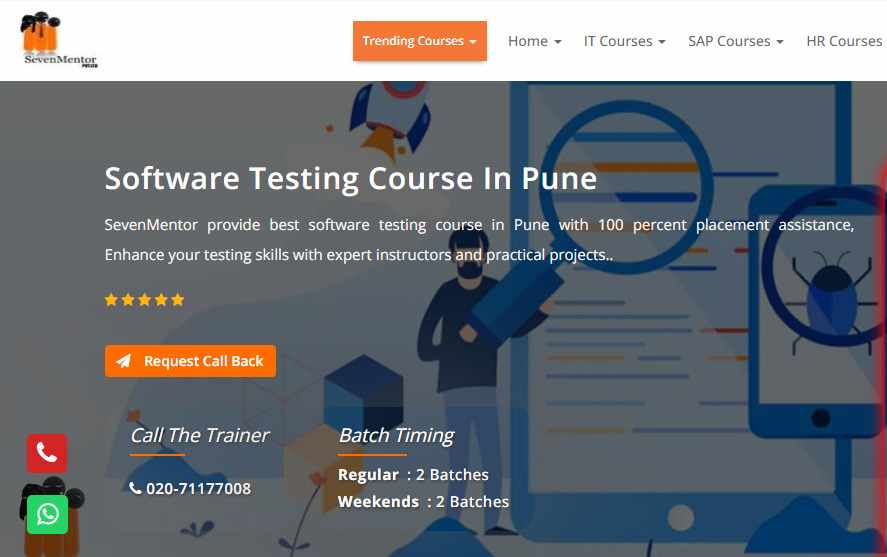
In the realm of software development, the integration of Artificial Intelligence (AI) has brought about transformative changes, particularly in the domain of software testing. AI-powered testing tools and methodologies have emerged as promising solutions to enhance efficiency, accuracy, and speed in the testing process. However, as with any disruptive technology, the rise of AI in software testing prompts a critical inquiry: Is it a boon or a bane? This article delves into the intricacies of AI in software testing, exploring its potential benefits and challenges while addressing concerns regarding its impact on the industry. Visit - Software Testing Classes in Pune
Boon: Enhancing Efficiency and Accuracy
One of the primary advantages of integrating AI into software testing is its ability to enhance efficiency and accuracy. Traditional testing methods often rely on manual efforts, which can be time-consuming, labor-intensive, and prone to human error. AI-powered testing tools, on the other hand, leverage machine learning algorithms to automate various aspects of the testing process.
AI-driven test automation frameworks enable rapid execution of test cases across diverse platforms and configurations, significantly reducing the time required for testing cycles. Moreover, AI algorithms can analyze vast amounts of data to identify patterns, anomalies, and potential defects more effectively than human testers. This not only streamlines the testing process but also improves the accuracy of defect detection, leading to higher software quality.
Furthermore, AI-based predictive analytics can anticipate potential areas of risk and prioritize testing efforts accordingly. By intelligently allocating resources and focusing on critical functionalities, organizations can optimize their testing strategies and achieve better overall outcomes.
Boon: Scalability and Flexibility
Another significant advantage of AI in software testing is its scalability and flexibility. As software systems become increasingly complex and dynamic, traditional testing approaches struggle to keep pace with evolving requirements and timelines. AI-powered testing solutions offer scalability by adapting to the changing needs of software projects, whether it involves testing web applications, mobile apps, or IoT devices.
Moreover, AI-driven testing tools can simulate real-world scenarios and user behaviors, enabling comprehensive testing across diverse environments and usage conditions. This flexibility is particularly valuable in agile and DevOps environments, where rapid iterations and continuous integration demand agile testing practices.
By leveraging AI for testing, organizations can achieve greater test coverage, identify edge cases, and ensure robust software performance under various scenarios. This not only enhances the reliability and resilience of software applications but also accelerates time-to-market, giving businesses a competitive edge in today's fast-paced digital landscape. Visit - Software Testing Course in Pune
Bane: Skill Gaps and Dependency
Despite its potential benefits, the widespread adoption of AI in software testing poses certain challenges and concerns. One of the primary issues is the skill gap and dependency associated with AI-driven testing tools. While AI algorithms can automate many aspects of testing, they require specialized knowledge and expertise to configure, maintain, and interpret results accurately.
As organizations transition to AI-based testing methodologies, they may encounter difficulties in sourcing and retaining skilled professionals with the requisite expertise in AI, machine learning, and data analytics. Moreover, reliance on AI for testing could lead to complacency among testers, diminishing their critical thinking skills and domain knowledge.
Furthermore, the black-box nature of AI algorithms raises concerns regarding transparency and accountability in testing processes. Unlike traditional testing methods where human testers can inspect and validate test cases manually, AI-driven testing tools often operate as opaque systems, making it challenging to understand the underlying decision-making processes and identify potential biases or errors.
Bane: Ethical and Privacy Implications
Another critical aspect to consider is the ethical and privacy implications of AI in software testing. As AI algorithms analyze sensitive data and user interactions to improve testing outcomes, there is a risk of privacy breaches and data misuse. Organizations must ensure compliance with regulatory frameworks such as GDPR and CCPA to safeguard user privacy and mitigate the risk of data breaches.
Moreover, the use of AI in software testing raises ethical concerns related to algorithmic bias and discrimination. AI algorithms trained on biased datasets may inadvertently perpetuate existing biases or discriminate against certain user groups. It is imperative for organizations to implement measures for bias detection and mitigation to ensure fairness and equity in testing processes.
The rise of AI in software testing presents both opportunities and challenges for the industry. While AI-powered testing tools offer significant advantages in terms of efficiency, accuracy, scalability, and flexibility, they also pose risks related to skill gaps, dependency, ethical considerations, and privacy implications. To harness the full potential of AI in software testing and mitigate its drawbacks, organizations must invest in talent development, ensure transparency and accountability in testing processes, and prioritize ethical considerations and privacy safeguards. By striking a balance between innovation and responsibility, the integration of AI can indeed prove to be a boon for software testing, driving improvements in quality, productivity, and user satisfaction. Visit - Software Testing Training in Pune
Write a comment ...